SAFE AI Industrial Chair
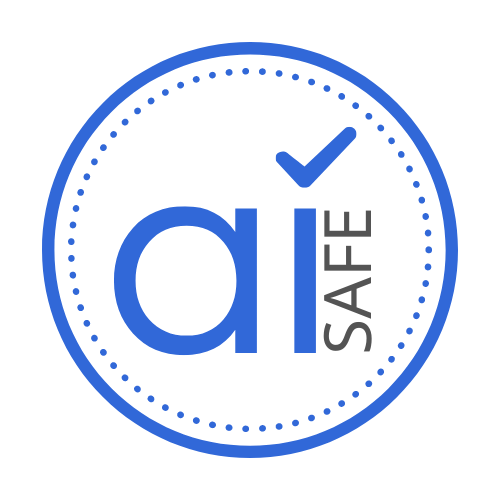
SAFE AI Industrial Chair
Prudent and Robust Learning for Safer Artificial Intelligence (SAFE AI)
Presentation

SAFE AI is a 5‑year research program led by its holder, Sébastien Destercke (CNRS Researcher at the Heudiasyc laboratory of UTC), the UTC Foundation for Innovation, SOPRA STERIA, the University of Technology of Compiègne, CNRS, and SCAI (The Sorbonne Center for Artificial Intelligence).
Theme
The chair is centered on Trusted AI (SAFE AI in English), specifically on reliable and robust AI.
The concept of Trusted AI encompasses several elements: transparency, ethics, explainability, and finally safety and robustness. In essence, reliable and robust AI involves quantifying the uncertainty of predictions, models, and data to ensure the reliability of AI systems. This is crucial in many industrial and social contexts: detecting machining defects, obstacles in autonomous transportation, medical conditions of patients, etc.
The chair aims to ensure a continuum between academic research and practical applications. To achieve this goal, in addition to the Heudiasyc laboratory, specialized in uncertain reasoning and intelligent autonomous transportation, and the LMAC laboratory, specialized in applied mathematics, the chair collaborates with three laboratories focusing on application fields related to the scientific project of the chair.
These laboratories are Roberval, specialized in mechanics and industry 4.0; BMBI specialized in biomechanics and e‑health; Avenues specialized in smart cities and urban engineering.
As part of the chair’s program, a research engineer will be recruited, whose main mission will be to implement case studies leading to prototyping, and possibly beyond.
One of the indicators of success in the long term will be the creation of an engineering hub or startups resulting from these efforts. Ultimately, this creates a beneficial ecosystem for everyone involved.
Research topics
The chair combines upstream research actions to develop new AI tools capable of addressing existing or future problems encountered in their application, with implementation and innovation actions on case studies.
Axis 1: Safe and Reliable Predictions
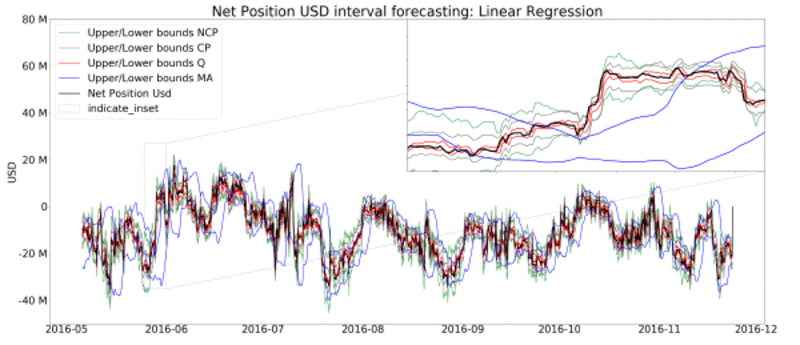
The challenge of this research axis is to make predictions with guaranteed error rates, aiming to increase confidence in the models and move towards their certification.
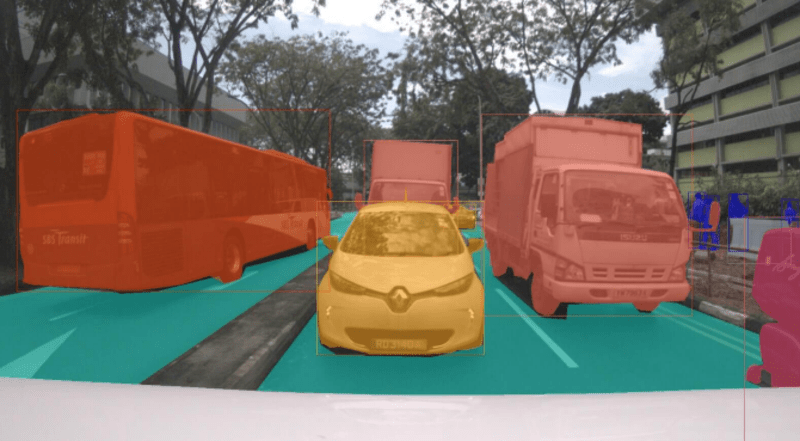
In particular, this axis focuses on providing such guarantees for each individual, rather than on average, and producing them in complex prediction spaces (problems including a temporal dimension, images, etc.).
Keywords
- Calibration
- Statistical guarantees
- Uncertainty quantification
- Conformal prediction
- Learning with abstention
Explored Application Domains
- Industry 4.0 (defect prediction)
- Autonomous transportation (obstacle recognition)
- Smart cities and energy (prediction of future consumption)
- Healthcare (medical diagnosis)
Axis 2: Robust Models
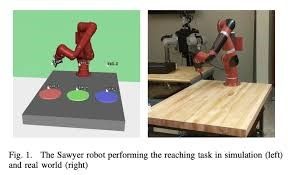
The challenge of this research axis is to obtain models robust to imperfect available data (« small and bad » data rather than « big ») or to the fact that the deployment environment differs from the model’s learning environment. For example, when transitioning from simulation (in-silico) or controlled environment (in-vitro) to a real environment (in-vivo), or when new classes not present during training appear.
Keywords
- Transfer learning
- Robust optimization
- Self-learning
- Optimal transport
- Missing or partial data
- Anomaly/detection novelty
Explored Application Domains:
- Autonomous driving and drones (simulation to real-world control)
- Healthcare (patient-specific models)
- Industry 4.0 (detection of new defects)
Axis 3: Collaborative Learning
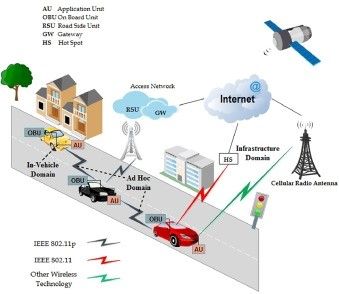
The challenge of this axis is to improve model quality, either through model-model collaboration (e.g., models relying on different modalities or measurements) or through model-human collaboration (by soliciting expert input in a relevant and limited manner).
Keywords
- Co-learning
- Self-learning
- Active learning
- Classifier fusion
Explored Application Domains:
- E‑health (smart home)
- Smart city and transportation (multiple sensors with possible failure/absence).
Équipe
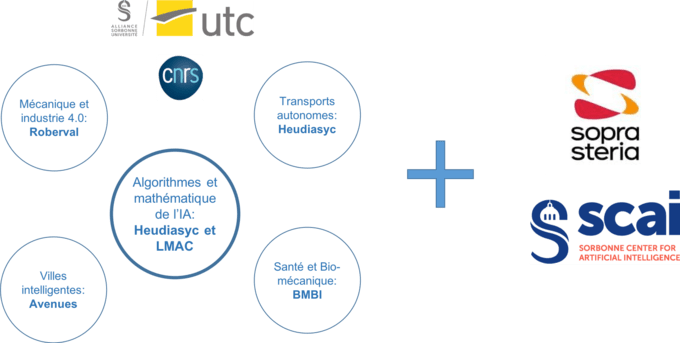
Le porteur scientifique de la chaire SAFE IA est Sébastien Destercke, chargé de recherche CNRS au laboratoire Heudiasyc de l’UTC (UMR UTC CNRS 7253).
Sa spécialité : la quantification d’incertitudes et le raisonnement dans l’incertain, notamment dans les données et l’IA. Il travaille au sein du laboratoire Heudiasyc (Heuristique et Diagnostic des Systèmes Complexes-UMR CNRS – 7253) qui opère dans le domaine des sciences de l’information et du numérique, notamment l’informatique, l’automatique, la robotique et l’intelligence artificielle.
La chaire associe également plusieurs enseignants-chercheurs des laboratoires de recherche de l’UTC :
Partners
SAFE AI brings together 5 partners:
- UTC Foundation for Innovation
- SOPRA STERIA, founding patron of the UTC Foundation for Innovation
- SCAI (The Sorbonne Center for Artificial Intelligence)
- CNRS (French National Center for Scientific Research)
- UTC (University of Technology of Compiègne)

News
CONTACT
Porteur scientifique | Sébastien Destercke
Tél : 03 44 23 79 85
Mail : sebastien.destercke@hds.utc.fr